Resources › eBook › Trust through transparency the choices you make as a leader in ethical ai › Why we use explainable rule based models
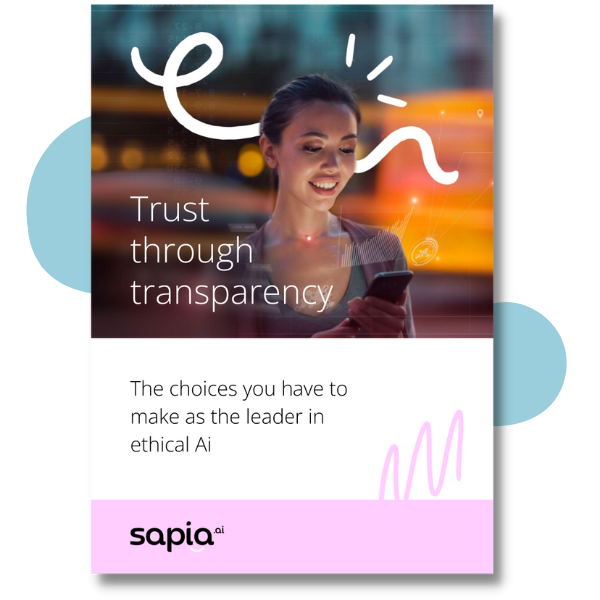
Why we use explainable rule-based models
We use explainable rule-based models, and not classical machine learning models, for scoring candidates.
Why does it matter? A rule-based model ensures a human is in the loop right from the outset. It enables the employer to know, and share internally, exactly what the technology is looking for. This is very different to the standard approach, which involves building a ML model from a historical dataset of hires. Historical data is problematic because it usually comprises a high performing group of hires, leading to a model that, for example, learns latent patterns associated with high performers compared to low performers.
That is when you risk amplifying historical biases in hiring, and when explainability becomes practically impossible. Not to mention that by building models off the performance variations in the already hired people, you are working with a restricted or filtered sample (referred more technically as having a restriction of range) that is not representative of the true candidate population. This can lead to machine learning models that can miss out on talent.
Out of all the product decisions we have made, this is by far the most important one to reduce bias and increase explainability in the use of AI models. There is a misconception in the market that to have an AI model, you must inevitably rely on a historical ‘people’ dataset. Our approach does not. We believe hiring is first and foremost a “human affair”.
Hiring organisations and managers have a candidate profile in mind that is aligned to the role requirements, which should not be totally ignored. In our approach, hiring managers and Sapia IO psychologists map those requirements to a set of rules that can discover talent with strict bias testing in place from model building to on-going scoring.
Bias testing against a norm group can reveal potential biases of using the rule set (e.g. gender bias) removing the risk of going live with a biased model (See our FAIR Framework for a detailed description of how we uphold fairness). The outcomes from bias testing can also inform how the rules can be adjusted to mitigate the discovered biases. This process also offers a great learning experience for hiring managers in understanding how some expected attributes of candidates can lead to biased outcomes.
The initial rules can also be optimised as hiring outcomes and further job performance data come to life. But unlike in building black box ML models, the whole process is transparent where all parties involved go through a data-driven decision making process learning and taking ownership of the scoring algorithm.
We see it as a form of “open sourcing” the development of the scoring algorithm with all the stakeholders involved.